한재덕 학생 IEEE Transactions on Image Processing 에 논문 게재 확정
2018.11.15 15:20
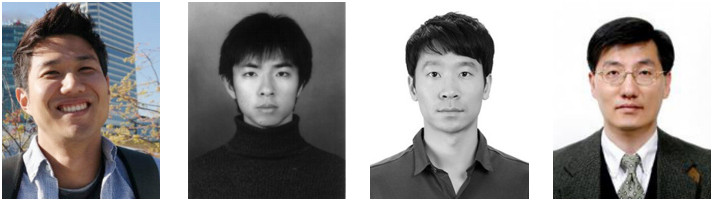
본 연구실의 통합과정 한재덕 학생이 연구한 “Permuted coordinate-wise optimizations applied to Lp-regularized image deconvolution” 제목의 논문이 영상처리분야 최고 권위의 학술지인 IEEE Transactions on Image Processing (Impact factor : 6.790, Rank : Q1) 에 2018년 7월 게재가 확정되었다. 본 연구는 연세대학교 전기전자공학과 통합과정 한재덕 학생(1저자), 통합과정 송기선 학생(2저자), 통합과정 김종현 학생(3저자)과 강문기 교수(교신저자)가 진행하였다.
Abstract
Image deconvolution is an ill-posed problem thatusually requires prior knowledge for regularizing the feasiblesolutions. In the literature, iterative methods estimate an intrinsic image, minimizing a cost function regularized by specific priorinformation. However, it is difficult to directly minimize the constrained cost function, if a nondifferentiable regularization (e.g.,the sparsity constraint) is employed. In this paper, we proposea nonderivative image deconvolution algorithm that solves the under-constrained problem (i.e., a non-blind image deconvolution) by successively solving the permuted subproblems. The subproblems, arranged in permuted sequences, directly minimize the nondifferentiable cost functions. Various L p-regularized (0 < p ≤ 1, p = 2) objective functions are utilized to demonstrate the pixel-wise optimization, in which the projection operator generates simplified, low-dimensional subproblems for estimating each pixel. The subproblems, after projection, are dealt within the corresponding hyperplanes containing the adjacent pixels of each image coordinate. Furthermore, successively solving the subproblems can accelerate the deconvolution process with a linear speedup, by parallelizing the subproblem sequences. The image deconvolution results with various regularization functionals are presented and the linear speedup is also demonstrated with a parallelized version of the proposed algorithm. Experimental results demonstrate that the proposed method outperforms the conventional methods in terms of the improved signal-to-noise ratio and structural similarity index measure.
댓글 0
번호 | 제목 | 글쓴이 | 날짜 | 조회 수 |
---|---|---|---|---|
65 |
김민섭 학생 IEEE Access 에 논문 게재 확정
![]() | 관리자 | 2018.12.22 | 4241 |
» |
한재덕 학생 IEEE Transactions on Image Processing 에 논문 게재 확정
![]() | 관리자 | 2018.11.15 | 1223 |
63 |
김민섭 학생 BK21플러스 사업 참여대학원생 수기 선정
![]() | 관리자 | 2018.11.08 | 1004 |
62 | 교수님방 및 연구실 이사 | 관리자 | 2018.03.12 | 6274 |
61 |
대한전자공학회 '논문상' 수상
![]() | 관리자 | 2014.12.02 | 1337 |
60 |
스승의 날
![]() | 관리자 | 2014.05.15 | 6370 |
59 |
홈페이지 리뉴얼
![]() | 관리자 | 2014.04.02 | 1518 |
58 | BK21 플러스 BEST 정보기술 사업단 세미나 개최 안내 | 관리자 | 2014.03.27 | 1472 |
57 | 연구실 동계 MT | 관리자 | 2014.03.27 | 1671 |
56 | 신입생 입학 | 관리자 | 2014.03.27 | 1543 |
55 |
BK21 TMS 정보기술 사업단 세미나 개최 안내
![]() | 관리자 | 2013.01.16 | 1318 |
54 |
BK21 TMS 정보기술 사업단 세미나 개최 안내
![]() | 관리자 | 2012.11.22 | 1282 |
53 |
[12/11/16] 바이오 영상 및 의공학 Workshop
![]() | 관리자 | 2012.11.15 | 1168 |
52 |
[12/11/08] 전기전자공학부 지도교수 간담회
![]() | 관리자 | 2012.11.15 | 1192 |
51 |
[12/11/08] EE-festival
![]() | 관리자 | 2012.11.14 | 1137 |
50 |
[12/10/19] 관측/촬영 MT
![]() | 관리자 | 2012.11.14 | 1224 |
49 |
"과학의 날" 우수과학자 초청 청와대 오찬 간담회
![]() | 관리자 | 2011.04.25 | 1576 |
48 | [8/28] 제59회 BERC 초청세미나 안내 | 관리자 | 2009.08.28 | 21887 |
47 | [8/3] 제58회 BERC 초청세미나 안내 | 관리자 | 2009.08.03 | 23014 |
46 | [7/23] srip 세미나 안내 | 관리자 | 2009.07.23 | 2106 |